Supercharge Your SDR Efficiency with AI-Driven Response Prioritization
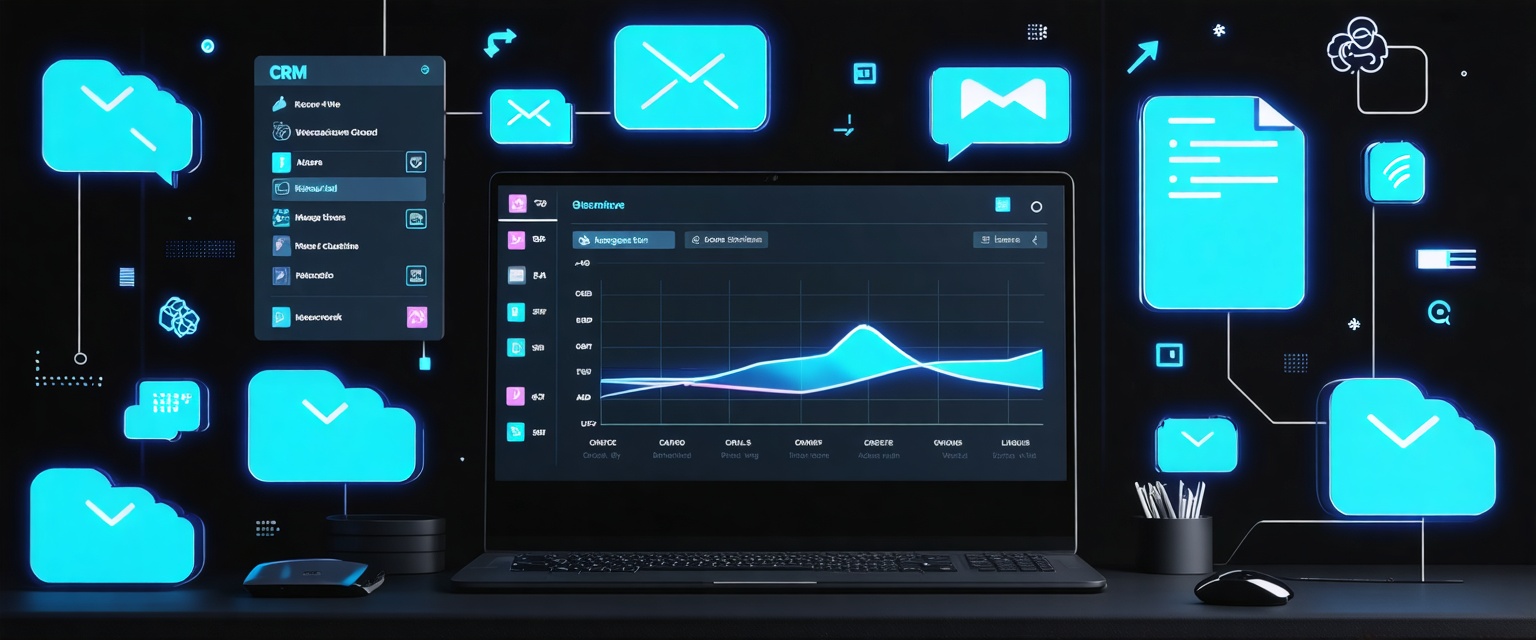
Explore how AI-driven response prioritization empowers SDRs, automating lead scoring and follow-ups, minimizing manual tasks, and maximizing conversion rates.
SDRs waste hours sifting through emails, leads, and messages trying to guess which ones deserve attention. Without clear signals, high-potential prospects get overlooked while low-priority ones eat up time.
Thanks to Agentic AI, automating response prioritization is now easier than ever. AI agents can instantly surface the most promising responses, allowing SDRs to focus on the right conversations.
Datagrid’s data connectors plug into existing systems so AI agents can surface high-priority responses in real time. This article explores how AI agents help SDRs automate response prioritization and focus on leads that actually convert.
Understanding Response Prioritization in SDR Workflows
Response prioritization involves systematically evaluating and ranking incoming leads and outbound responses to ensure the most promising opportunities receive timely attention. For SDRs juggling dozens or hundreds of potential conversations daily, this process determines which prospects get immediate follow-up and which wait in line.
Core Tasks Involved in Response Prioritization
- Lead intake: Capturing new leads from various sources like website forms, email inquiries, and social media, which can be optimized using AI for lead generation
- Initial qualification: Quickly assessing basic fit criteria like company size, industry, and budget
- Lead scoring: Assigning numerical values based on engagement level, firmographics, and behavioral signals
- Urgency assessment: Determining which leads require immediate follow-up versus those that can wait
- Queue management: Organizing leads into appropriate follow-up sequences or assigning them to specific SDRs
This means checking CRM updates for new leads, verifying job titles on LinkedIn, and prioritizing responses to those who've recently engaged with your content.
How Response Prioritization Drives Sales Productivity
Effective response prioritization directly impacts key sales performance indicators:
- Shorter sales cycles: By focusing on the most qualified and engaged leads, SDRs move prospects through the pipeline more quickly
- Improved conversion rates: Prioritizing high-potential leads increases the likelihood of successful conversions
- Better pipeline management: A structured approach to prioritization ensures a steady flow of quality leads to account executives
For revenue teams, this means more predictable forecasting, improved resource allocation, and increased sales productivity.
Typical Data Inputs for Prioritization Decisions
Sales Development Representatives rely on various data points to make informed prioritization decisions:
- Demographic data: Job title, company size, industry
- Firmographics: Annual revenue, growth rate, technological stack
- Engagement history: Email opens, website visits, content downloads
- Recent activity: Attendance at webinars, requests for demos
- Intent signals: Searches for competitor names, visits to pricing pages
Picture this: a lead who opened three emails in the past 24 hours and visited your pricing page ranks higher than someone who downloaded a whitepaper two weeks ago but hasn't engaged since.
What Slows Down SDRs During Response Management?
Despite understanding the importance of rapid, strategic lead prioritization, most SDRs struggle with several bottlenecks that drain their productivity and effectiveness.
Data Gathering and Lead Research
The constant tab-switching dance between LinkedIn profiles, CRM records, company websites, and other platforms creates massive inefficiency. SDRs spend precious minutes copy-pasting job titles or company information from various sources into their CRM, leading to fragmented and often outdated lead profiles that require regular prospect database cleanup.
Lead Scoring and Qualification Delays
Manual lead scoring introduces inconsistency and subjectivity that slows down prioritization:
- Different SDRs interpret scoring criteria differently, creating inconsistent lead prioritization
- Personal biases influence scoring, causing high-value leads to be overlooked
- Checking various data points for each lead delays response times
- Inconsistent application of qualification criteria leads to pipeline quality issues
An SDR might waste valuable minutes debating whether a lead deserves a high or medium score while clear opportunities wait in the queue.
Missed Buying Signals and Poor Timing
Timing is everything in sales, yet manual monitoring of lead activities results in missed opportunities.
A prospect might view a pricing page multiple times but not receive a follow-up call until days later. Similarly, a lead could download a case study indicating high interest, but the SDR doesn't notice until after their weekly review.
The odds of qualifying a lead drop dramatically if follow-up doesn't occur within the first hour of a prospect's initial engagement.
Administrative Overhead and Workflow Fragmentation
SDRs lose momentum and focus through constant context-switching:
- Updating CRM records after each interaction
- Logging call notes and email summaries
- Scheduling follow-up tasks and reminders
- Coordinating with marketing for additional lead information
This fragmentation not only slows down response management but increases the risk of human error in data entry and follow-up scheduling.
How AI Agents Automate Response Prioritization for SDRs
AI agents transform how SDRs manage and prioritize responses by handling the data-heavy, repetitive tasks that slow down human reps. Here's exactly how they work:
Automated Data Aggregation and Enrichment
AI agents pull data from multiple sources simultaneously, creating comprehensive lead profiles in seconds, effectively automating lead enrichment.
They extract company details from the CRM, pull professional background from LinkedIn, gather technographic data, and compile engagement history across email, website, and social channels.
This eliminates the tab-switching nightmare SDRs face daily.
Machine Learning-Based Lead Scoring and Ranking
AI scoring models evaluate leads using patterns learned from historical conversion data:
- Analyzing which lead characteristics correlated with past sales
- Weighting factors differently based on their predictive power
- Applying consistent criteria across all leads
- Producing numerical scores that enable objective ranking
Unlike static, rules-based scoring systems, AI models continuously refine their approach based on which leads actually convert.
Real-Time Signal Monitoring and Trigger Detection
AI agents constantly scan for buying signals across multiple channels, including website behavior, email engagement, content interactions, and external triggers like funding announcements or leadership changes.
When significant signals appear, AI immediately reprioritizes the lead and alerts the SDR. This ensures follow-up happens while interest is highest.
Automated Outreach and Follow-Up Sequencing
Based on lead scores and behaviors, AI agents can draft personalized initial outreach messages, schedule follow-ups at optimal times, suggest talking points based on prospect interests, and recommend next steps when conversations stall. These capabilities significantly enhance sales engagement, leading to better conversion rates.
AI-powered prioritization identified the top leads that deserved immediate attention, directing sales efforts where they mattered most and substantially increasing conversion rates.
Continuous Learning and Prioritization Refinement
The true power of AI agents emerges over time as they learn from outcomes.
They adjust scoring weights based on which factors actually predict sales, recognize changing market conditions, identify new patterns that human reps might miss, and evolve prioritization logic without requiring manual updates.
Businesses achieve a major increase in conversion rates using AI for lead prioritization, with the system becoming more accurate with each sales cycle.
Datagrid for Sales Professionals
Sales teams constantly juggle prospect information, deal documentation, and competitive intelligence across multiple systems. Datagrid's AI-powered platform delivers specialized solutions to accelerate your sales cycle.
Lead Intelligence Enhancement
Process thousands of prospect interactions, company data points, and market signals simultaneously to identify high-potential opportunities and optimal engagement timing. Datagrid helps you see the complete picture of each lead in seconds rather than minutes of manual research.
Sales Document Automation
Extract critical information from RFPs, proposals, and contracts to generate customized sales materials that address specific client needs without starting from scratch. Sales reps using Datagrid report saving 5-7 hours weekly on document preparation alone by automating sales proposals.
Competitive Win/Loss Analysis
Deploy AI agents to analyze won and lost deals across your CRM, identifying patterns in decision criteria, objection types, and competitive positioning that influence closing rates. This intelligence helps your team focus on deals they're most likely to win and adjust strategy for challenging competitive situations.
Client Communication Intelligence
Automatically analyze email exchanges, meeting notes, and call transcripts to identify buying signals, stakeholder sentiments, and potential roadblocks requiring attention. Datagrid detects nuances and context that help SDRs tailor their approach to each stakeholder's concerns.
Pipeline Health Monitoring
Process historical deal data to predict close probabilities more accurately, identify stalled opportunities, and recommend specific actions to advance stagnant deals. Sales managers using Datagrid get early warning of at-risk deals and concrete suggestions for getting them back on track.
Simplify Sales Tasks with Datagrid's Agentic AI
Don't let data complexity slow down your team. Datagrid's AI-powered platform is designed specifically for teams who want to:
- Automate tedious data tasks
- Reduce manual processing time
- Gain actionable insights instantly
- Improve team productivity
See how Datagrid can help you increase process efficiency.